As Large Language Models (LLMs) continue to revolutionize various industries, their impact on mental health applications has been both promising and challenging. At Lua Health, we've been diligently evaluating the strengths and limitations of LLMs in the realm of mental health since the advent of ChatGPT. Our AI team has recently published their work to help other researchers understand the limitations of LLM in the mental health field.
LLMs are a category of artificial intelligence (AI) models designed to understand and generate human-like language. These models, such as OpenAI's GPT-3.5, have gained widespread attention for their ability to comprehend context, generate coherent text, and perform a range of language-related tasks. In the mental health sector, LLMs have shown promise in processing and analyzing written language to identify potential signs of emotional distress or mental health disorders.
Traditional Machine Learning (ML) methods, on the other hand, rely on algorithms and statistical techniques to make predictions based on data patterns. These methods have been employed in various applications, including mental health, and have established themselves as reliable tools for analyzing structured and unstructured data.
At Lua Health, we conducted a range of experiments to compare the effectiveness of LLMs and traditional ML methods in predicting depression and anxiety from written language. We used a range of closed and open source LLMs, and traditional ML algorithms.
Surprisingly, our experiments revealed that traditional ML methods still outperformed LLMs in predicting depression and anxiety. The traditional algorithms demonstrated a higher accuracy in identifying subtle linguistic cues indicative of emotional distress, showcasing their continued relevance in the mental health domain.
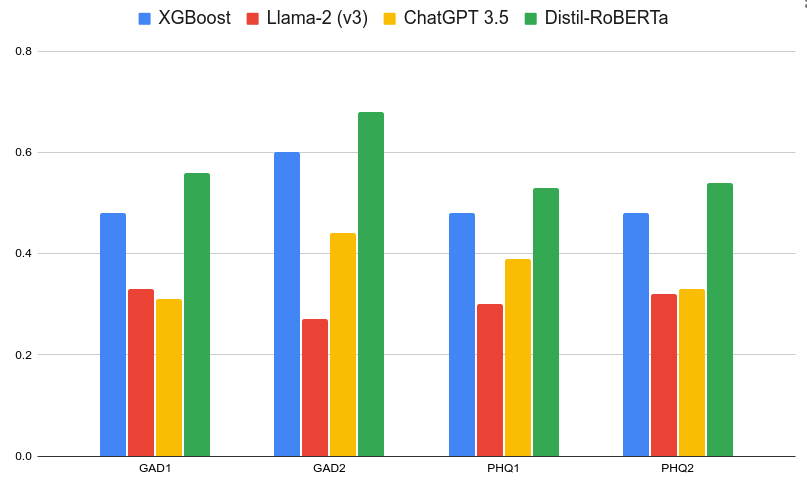
The full details of the experiment can be found in the team's arxiv paper. We hope by sharing our research with the wider world, we together can improve the global burden of mental health.
While LLMs excel in understanding context and generating human-like responses, they may struggle with the nuanced subtleties present in mental health-related language. Traditional ML methods, with their focus on pattern recognition and statistical analysis, proved to be more adept at discerning these intricate linguistic nuances, leading to superior predictive accuracy.
The integration of AI, particularly LLMs, in mental health applications holds immense promise. However, as our experiment at Lua Health indicates, there are situations where traditional ML methods still outshine their newer counterparts. Striking a balance between the strengths of LLMs and traditional ML approaches may be the key to unlocking the full potential of AI in mental health diagnostics and treatment.
As technology continues to evolve, Lua Health remains committed to exploring innovative solutions that leverage the strengths of both LLMs and traditional ML methods to provide comprehensive and accurate mental health assessments. Our journey in navigating the intersection of AI and mental health continues, driven by a commitment to improving the well-being of individuals through cutting-edge technology.